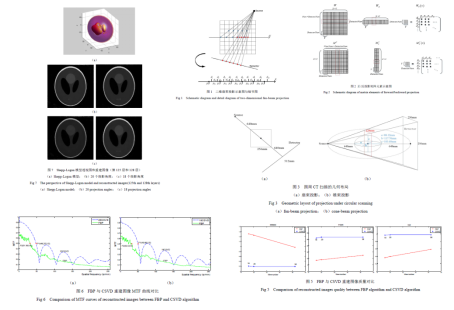
Studies have shown that X-ray radiation from CT examinations increases the risk of cancer in patients. But the risk can be reduced by virtue of decreasing the number of projection view angles. So it is of great clinical significance to study how to reconstruct CT images from sparse projection data. Using the compressed sensing (CS) theory, we have proposed some novel algorithms for recent years so as to reconstruct images from sparse CT projection data with sufficient accuracy (e.g., (a) Limited View Reconstruction for Differential Phase-contrast Computed Tomography, Optics Express. 2015, 23(8): 9717-9729; (b) An Iterative Image Reconstruction for Differential X-ray Phase-contrast Computed Tomography, Journal of Fiber Bioengineering and Informatics, 2015, 8(3): 521-528). It means the CT images reconstructed by our methods are suitable for clinical diagnosis.
In our latest work ((a) View-driven Compressed Sensing Method of CT Image Reconstruction, IOP Conf. Ser.: Mater. Sci. Eng. 768 (2020) 062003 062003; (b) CT Image Reconstruction from Ultra-sparse Projection Data (in Chinese), Journal of Beijing University of Aeronautics and Astronautics, 2020), the numerical simulations show that our algorithm has high numerical accuracy, low computational complexity, less memory overhead, and strong engineering practicability under the condition of ultra-sparse projection data (i.e., no more than 20 projection view angles for fan-beam/cone-beam CT scanning in the range of ). Currently, we are looking for cooperation with the industry towards the commercialization.