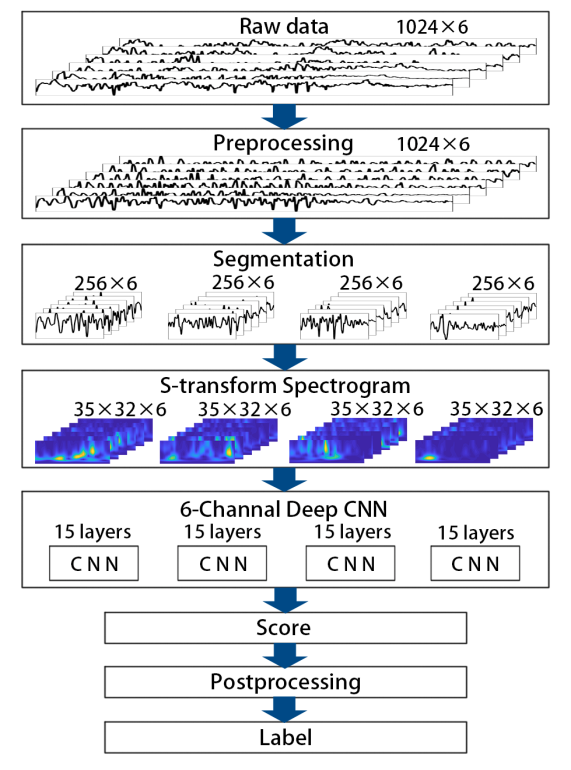
Automatic seizure detection is significant for the diagnosis of epilepsy and reducing the massive workload of reviewing continuous EEGs.In this work, a novel approach, combining S-transform with deep Convolutional Neural Networks (CNN), is proposedto detect seizure onsets in long-termintracranialEEG recordings.Primarily, raw EEG data is filtered with wavelet decomposition. Then a fine-tuned Stockwell transform (S-transform) is used to obtain a proper time-frequency representation of each EEG segment. After that, a 15-layer deep CNN using dropout and batch normalization serves as a robust classifier. Finally, smoothing, collar technique and fusion decision are applied to the outputs of CNN to improve the detection accuracy and reduce thefalsedetectionrate(FDR).
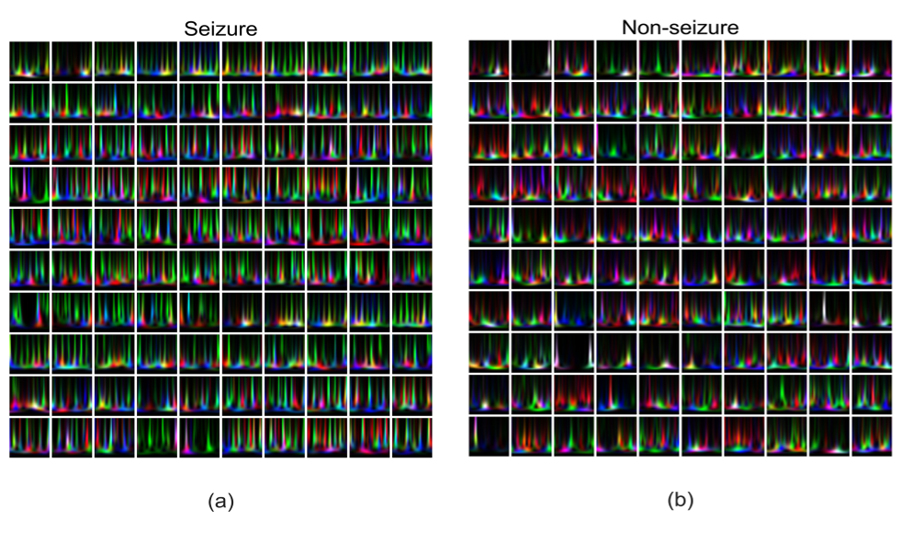
The segment-based and event-based evaluationassessmentsand ROC curves are employed for theperformance evaluationon a public EEG database containing 21 patients. A segment-based sensitivity of 97.01% and a specificity of 98.12% are yielded. For the event-based assessment, this method achieves a sensitivity of 95.63% with a false detection rate of 0.36/h.[ Guoyang Liu, Weidong Zhou*, Minxing Geng. Epileptic Seizure Detection System Based on S-Transform Spectrogram and Deep Convolutional Neural Network,International Journal of Neural Systems, 29,1950024-1950039, 2019 ]